Alcohol use causes 88,000 deaths in America each year and results in a $250 billion annual economic burden due to fatalities, medical expenditures, and costs associated with loss of workplace productivity1,2. As such, a number of animal models have been used to recapitulate the physiological and functional outcomes of alcohol use disorder (AUD) with rodent models being the most widely studied3,4. However, the anatomical differences between rodents and humans pose a number of limitations in studying the complex nature of human AUD. For example, rodents generally do not voluntarily consume alcohol to intoxication and once consumed, rodents metabolize alcohol much faster than humans3. These characteristics make modeling chronic intoxication and physiological responses challenging. In contrast, our porcine model of AUD possesses great translational potential with comparable consumption and intoxication patterns, metabolism, and organ anatomy relative to humans5-8. We believe pigs may serve as an ideal model system to advance human AUD research.
Pigs and humans share many anatomical similarities that allow for a more direct extrapolation of porcine AUD findings to humans. For example, pigs and humans share a similar rate of alcohol elimination by the liver7,9. The pig and human gastrointestinal tract is also comparable in terms of length, transit time, and surface area which dictate alcohol metabolism and absorption rates10,11. Shared gyrencephalic cerebral architecture and white matter volumes also enable more representative evaluations of susceptibility and neural tissue damage due to AUD12,13. Collectively, these metabolic and anatomical similarities between pigs and humans suggest our porcine AUD model could be highly predictive of human AUD14.
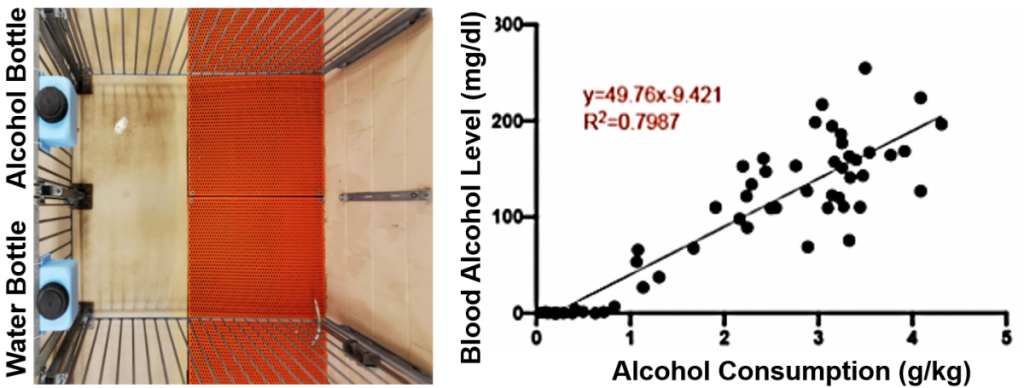
- 1Sacks, J.J., et al., 2010 National and State Costs of Excessive Alcohol Consumption. Am J Prev Med, 2015. 49(5): p. e73-e79.
- 2Mokdad, A.H., et al., Actual causes of death in the United States, 2000. JAMA, 2004. 291(10): p. 1238-45.
- 3Crabbe, J.C., R.A. Harris, and G.F. Koob, Preclinical studies of alcohol binge drinking. Ann N Y Acad Sci, 2011. 1216: p. 24-40.
- 4Fernandes, L.M.P., et al., Repeated Cycles of Binge-Like Ethanol Intake in Adolescent Female Rats Induce Motor Function Impairment and Oxidative Damage in Motor Cortex and Liver, but Not in Blood. Oxid Med Cell Longev, 2018. 2018: p. 3467531.
- 5Dexter, J.D., et al., Sinclair(S-1) miniature swine as a model for the study of human alcoholism. Ann N Y Acad Sci, 1976. 273: p. 188-93.
- 6Tumbleson, M.E.H., D. P.; Dexter, J. D.; Middleton, C. C., Miniature swine as a model for the study of human alcoholism: The withdrawal syndrome. Fermented Food Beverages in Nutrition, 1979: p. 457-475.
- 7Keiding, S., et al., Ethanol elimination kinetics in human liver and pig liver in vivo. Am J Physiol, 1979. 237(4): p. E316-24.
- 8Shin, S., et al., An Adolescent Porcine Model of Voluntary Alcohol Consumption Exhibits Binge Drinking and Motor Deficits in a Two Bottle Choice Test. Alcohol and Alcoholism, 2020.
- 9Paton, A., Alcohol in the body. BMJ, 2005. 330(7482): p. 85-7.
- 10Bode, G., et al., The utility of the minipig as an animal model in regulatory toxicology. J Pharmacol Toxicol Methods, 2010. 62(3): p. 196-220.
- 11Spurlock, M.E. and N.K. Gabler, The development of porcine models of obesity and the metabolic syndrome. J Nutr, 2008. 138(2): p. 397-402.
- 12Gralla, J., et al., A dedicated animal model for mechanical thrombectomy in acute stroke. AJNR Am J Neuroradiol, 2006. 27(6): p. 1357-61.
- 13Kobayashi, E., et al., The pig as a model for translational research: overview of porcine animal models at Jichi Medical University. Transplant Res, 2012. 1(1): p. 8.
- 14Shin, S. K., et al., Alcohol Induced Brain and Liver Damage: Advantages of a Porcine Alcohol Use Disorder Model. Frontiers in physiology, 2021. 11: p. 592950.