It’s estimated that approximately 20 million chickens in the U.S. die in commercial poultry houses before reaching market age or arriving at slaughterhouses for various reasons. In commercial broiler or cage-free laying hen houses, thousands of chickens are housed within the same facility in high density. Animal wellbeing monitoring, including daily mortality checks, is typically conducted manually, which is time-consuming and labor-intensive. The causes of chicken mortality can vary, and farm staff aim to identify these issues quickly in order to assess potential risks and prevent disease spread. In cage-free houses, locating dead chickens (Figure 1) can be particularly challenging due to the interference of equipment and other facilities. Leaving deceased chickens unattended can negatively impact animal welfare and overall health. As such, developing an automated system to continuously monitor and identify dead chickens in poultry houses is essential.
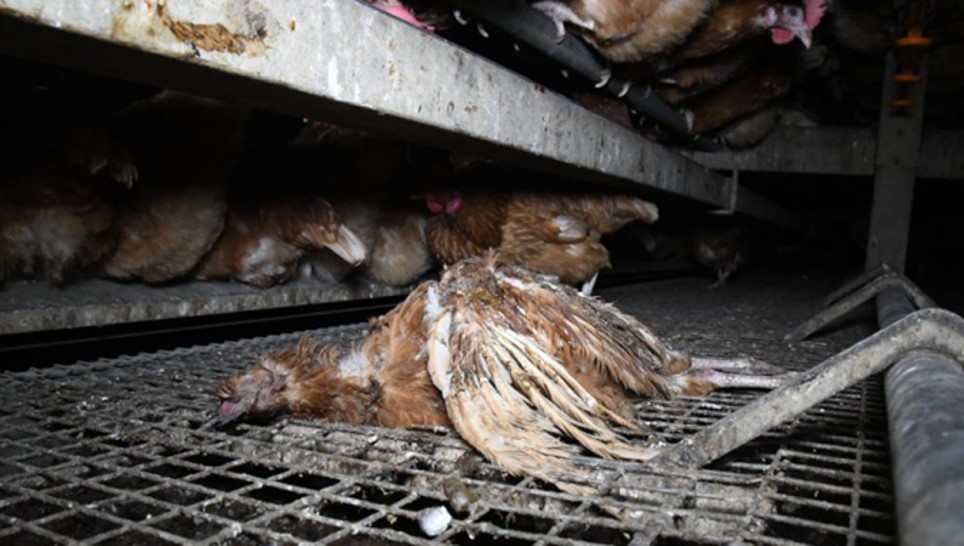
Figure 1. A dead chicken in cage-free aviary system (Photo Credit: Animal Aid).
Researchers at the University of Georgia (i.e., Dr. Lilong Chai’s lab in the Department of Poultry Science and Dr. Guoyu Lu’s lab in the College of Engineering) developed a robotic machine vision method based on “Go 1” Robot and YOLOv8 deep learning model recently. The method has been tested in research cage-free facilities for navigating dead chickens and floor eggs automatically (Figure 2). Bird and egg images were extracted from the robot dog and annotated using V7 Darwin, an online annotation tool provided by V7labs (V7, 8 Meard St, London, United Kingdom). This tool supports various formats, including JPG, PNG, TIF, MP4, MOV, SVS, DICOM, NIfTI, and more, enabling the consolidation of training data in one place.
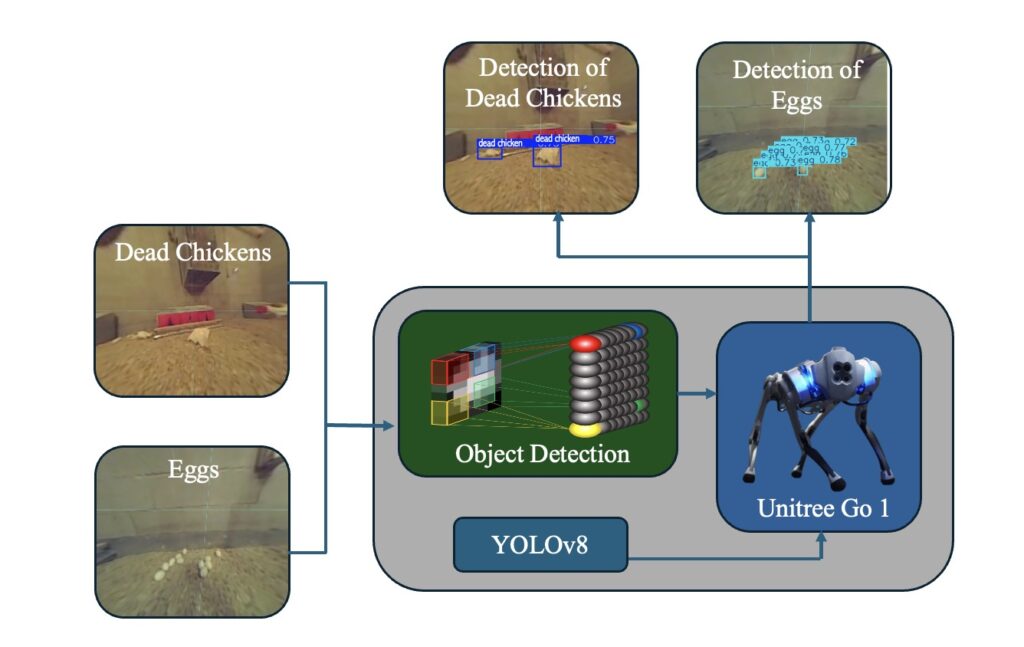
Figure 2. The “Go 1” Robot and YOLOv8 deep learning model for tracking dead chicken and floor eggs.
In this study, the team recorded the entire process of chickens’ interactions with a robotic entity over the course of one hour. The observation focused on the chickens’ initial reactions and subsequent behavior changes, documenting phases of fear, curiosity, intimacy, and normalization. The robot was positioned in our observation area, which included half a drinking line, two feeders, and one nesting box, representing a typical section of a cage-free house. The results show that the average accuracy of each detector ranges from 85% to 91%, with the best model being YOLOv8m, which achieved a precision of 91%. The detector can effectively recognize various floor eggs on the litter or under feeders and detect dead chickens in corners or around healthy chickens (Figure 3). This detector can be further combined with mechanical arms, such as soft suction mechanisms or soft rubber grippers, to pick up floor eggs. It can also be equipped with a secondary robot to remove dead chickens using location information provided by the robot.
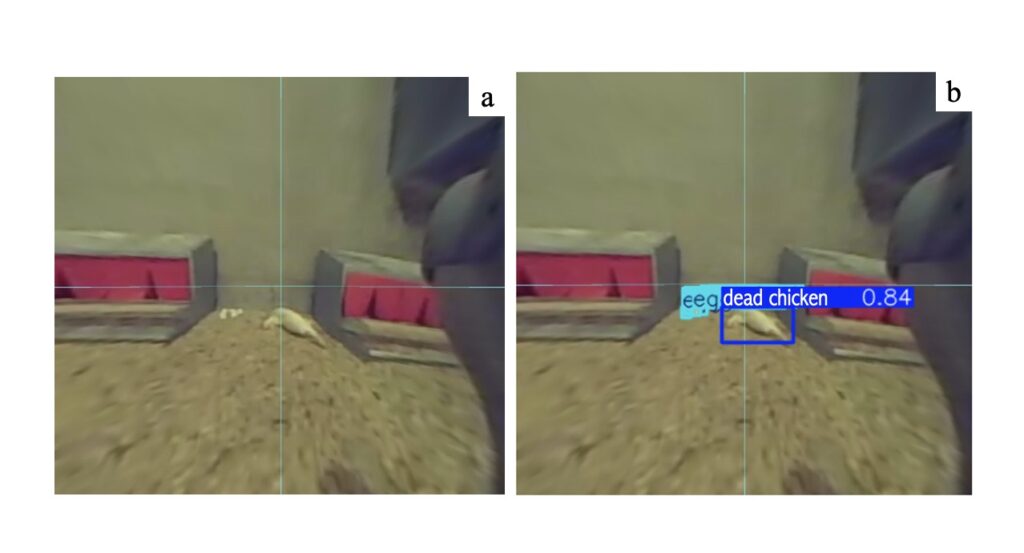
Figure 3. Dead chicken tracking in cage-free hen facilities.
In summary, a robotic machine vision method (Figure 4) was developed for tracking dead chickens based on “Go 1” Robot dog and YOLOv8 networks in cage-free facilities for this study. While the YOLOv8 model performed well in detecting dead chickens and floor eggs, there were some limitations. The robot struggled with detecting eggs in low light or when blocked by obstacles. Additionally, factors like flock density and occlusions could affect detection accuracy. The results provide an actionable approach to detecting floor eggs and dead chickens in cage-free houses using a single system without intrusion. This study demonstrates the potential of using intelligent bionic quadruped robots to address the issues of floor eggs and dead chickens in cage-free houses. These advancements provide valuable information for using robotics to help improve the management of cage-free chickens. In the future, the team plan to innovate imaging system with adjustable height for identifying dead chickens in aviary system.
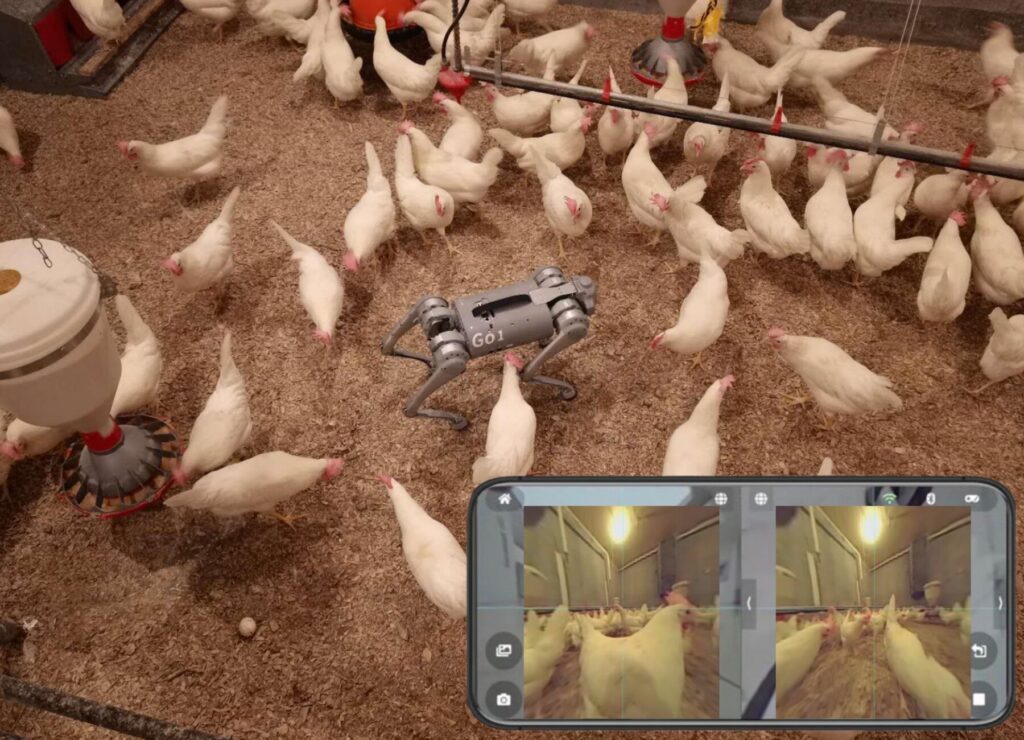
Figure 4. Robotic machine vision method for tracking dead chickens and floor eggs.
Further reading:
Yang, X., Zhang, J., Paneru, B., Lin, J., Bist, R. B., Lu, G*., & Chai, L*. (2025). Precision Monitoring of Dead Chickens and Floor Eggs with a Robotic Machine Vision Method. AgriEngineering, 7(2), 35. https://doi.org/10.3390/agriengineering7020035