Domestic fowl shows the tendon-locking phenomenon, which lets them to express perching behavior (PB) with minimum muscular effort. The domestic hens look for an elevated structure for resting during night as that the jungle fowl, in order to prevent themselves from ground predators. Providing perches in cage-free (CF) housing (Figure 1) offers significant benefits for laying hens, such as improved leg muscle development, bone health, reduced abdominal fat, and decreased fear and aggression. Hens show strong desire to use perches for roosting, showing their willingness to put an effort to gain an access to perches. Additionally, they exhibit signs of agitation and frustration when accessing perches is restricted or denied resulting into reduced welfare. However, most of cage-free hens have been reported with bone damages or fractures (e.g., keel and leg bones) due to failure in perching. Therefore, it is meaningful to monitor hens’ perching behaviors for improving perching design and animal welfare.
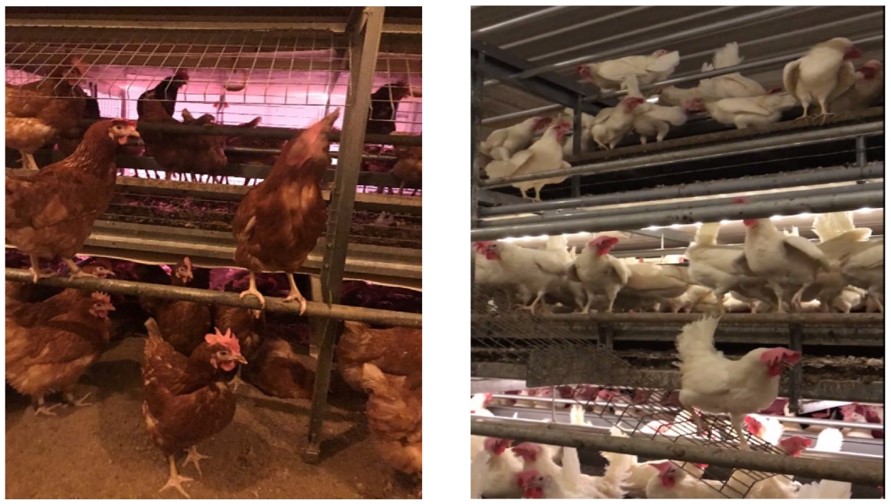
Fig. 1. Perching hens in cage-free houses.
Researchers at the University of Georgia’s poultry science department developed a machine vision system for monitoring hens’ perching behaviors in a research cage-free facility (Figure 2) automatically. Four identical research poultry facilities (rooms) were used, and 200 chicks (Hy-line W-36) were placed in each room from day 1 to day 525. To meet the CF housing guidelines, these rooms were designed with a provision of perches and litter floor. Each room were identical in terms of dimensions (7.3 meters in length, 6.1 meters in width, and 3 meters in height) and facility (feeders, drinkers, lighting, perches, and nest boxes). Pine shavings (~5 cm deep) was placed in each room as a bedding material from day 1. Environmental factors such as indoor temperature, relative humidity, light duration (16 hours), light intensity (12-15 lux), and ventilation rates were automatically regulated and recorded using the Chore-Tronics Model 8 controller.
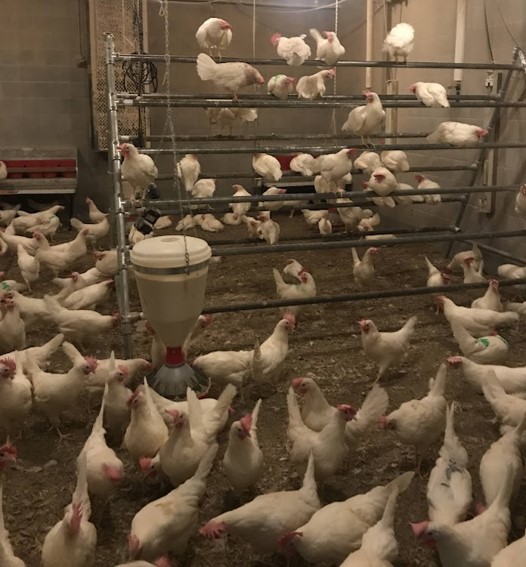
Fig. 2. Research cage-free facility housing pullets/laying hens.
Video datasets collected from research facilities were converted into individual image files in .jpg using Free Video to JPG Converter App (ver. 5.0) at 15 frame per second (FPS) image processing rate. Image datasets obtained were filtered and separated manually based on perching behavior presence in the images and high-quality image datasets. Five hundred images from manually separated images were randomly selected that included images from different days and different time within each growth phase of the birds resulting into total 3000 images. Out of 3000 images, 70% were used for training, 20% for validation, and 10% for testing. Selecting images from the same day and same time could limit the ability of the model to precisely detect perching behavior that occurs throughout the life period of the birds. Thus, we selected images randomly from different days and time within each growth phase. The detailed process of data collection, labeling, pre-processing, training, validation, testing, and implementation is shown in Figure 3. The YOLOv7 and YOLOv8 models we trained were originally from the GitHub repository developed by Ultralytics. All YOLOv7 and YOLOv8 models used in this study were pretrained with common objects in context datasets and can be readily modified into required object detection models through target object training datasets. Before developing the perching model detector, the experimental configurations were prepared for the model evaluation.
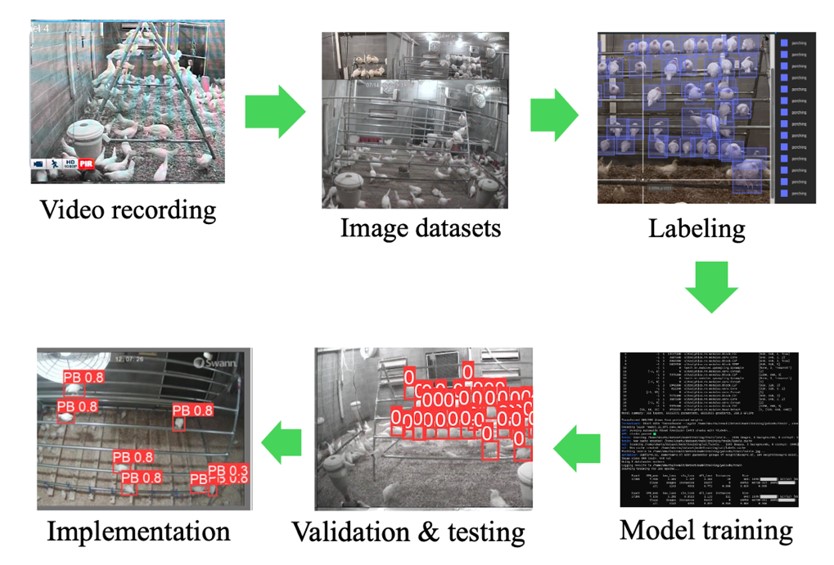
Fig. 3. The processes of perching detection (PD) system (i.e., data collection, labeling, training, validation, testing, and implementation).
Detection precision of perching behavior reached 94% for hens at different ages (Figure 4). From the tested images, comprehensive analysis confirms the remarkable performance of the YOLOv7x-PB & YOLOv8x-PB models in effectively detecting PB behavior. The YOLOv8x-PB model’s higher recall and mAP@0.50, mAP@0.50:0.95, and F1- scores collectively highlights its ability of detecting PB behavior within CF housing conditions. Each incremental improvement in performance metrics was important in reducing false detections by the model. Therefore, every margin increase in performance metric holds significance within the context of the detection model. These findings confirm the model’s potential as a valuable tool for speeding up prompt and correct PB detection, thereby enhancing the efficiency of poultry management practices not only applicable in CF housing but could be applicable in other housing conditions for broilers and laying hens.
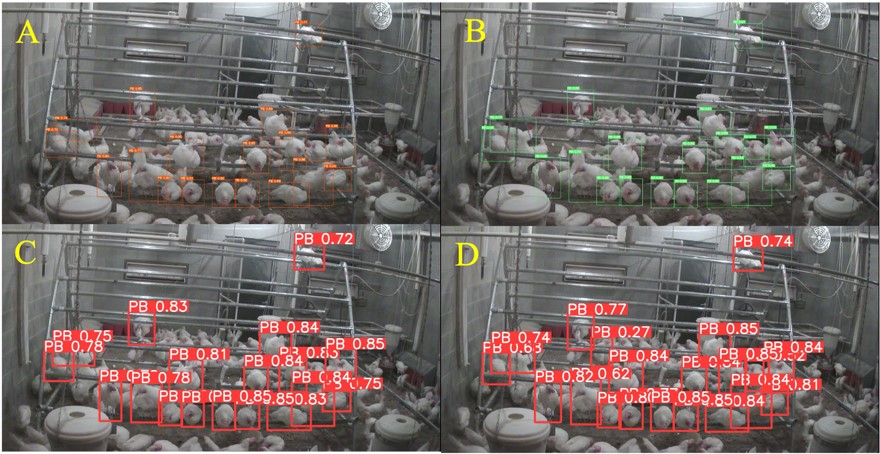
Fig. 4. Comparison of perching detection (PB) detection on the same image using four variants of YOLO models, where A= YOLOv7-PB model, B=YOLOv7x-PB model, C= YOLOv8s-PB model, D= YOLOv8x-PB model.
The study highlighted the benefits of utilizing the new addition of YOLO models, i.e. YOLOv8x-PB in accurately detecting PB behavior with higher precision (>94%). This finding provides a valuable reference for detecting PB behavior of cage-free hens for optimizing perch design and installation in commercial housing system.
Further reading: Paneru, B., Bist, R., Yang, X., & Chai, L*. (2024). Tracking perching behavior of cage-free laying hens with deep learning technologies. Poultry Science, 103(12), 104281.