High pathogenicity avian influenza (HPAI) is disastrous for poultry industries worldwide. The outbreak of HPAI in 2014-2015 caused the loss of 60 million chickens and turkey, and the most recent HPAI outbreak since 2021 has led to losses of over 100 million chickens so far in the US. Farm biosecurity management practices have been applied to prevent the spread of virus. However, there are no efficient strategies for preventing wild birds’ contact with animal farms. Wild birds, especially some waterflow and shorebirds are the natural hosts of avian influenza (AI) virus. They can be infected with HPAI and show no symptoms. Wild birds’ droppings spread AI virus to domestic flocks when migrating. AI virus are classified into two groups based on their ability to cause diseases: low pathogenic avian influenza (LPAI) and highly pathogenic avian influenza (HPAI). LPAI generally causes no signs of illness or minor symptoms in chickens. However, two strains of LPAI, the H5 and H7 strains can mutate into HPAI. HPAI has a higher mortality rate in chickens than LPAI, and spreads rapidly, even could be passed to humans.
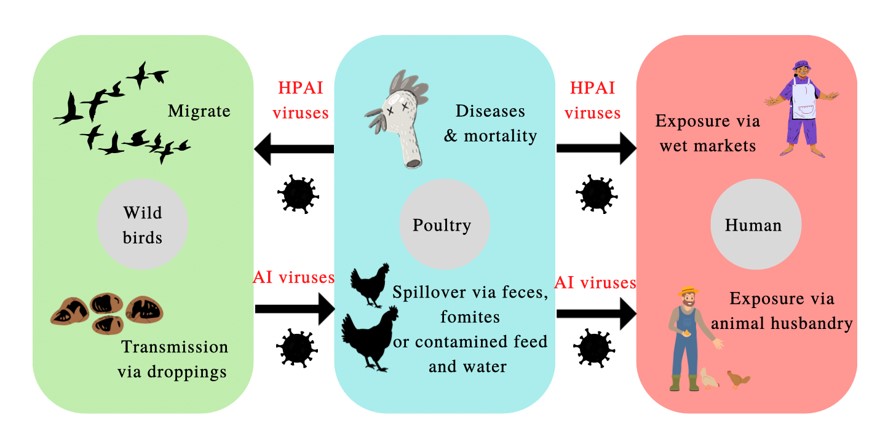
Figure 1. Generalized transmission of avian influenza viruses.
To address the potential technical gap in wild bird management, a machine vision system (Figure 2) was developed by researchers at the University of Georgia using regular 2D cameras and deep learning models. The system includes a 1080p full high-definition camera (PRO-1080MSFB) (the installation height of the camera is 1.5 m), a digital video recorder (DVR-5580), and a computer (Dell-P2419H) at the poultry research at the University of Georgia (UGA), Athens, GA, USA. Automated detection of wild birds provides an effective way to understand AI transmission pathway.
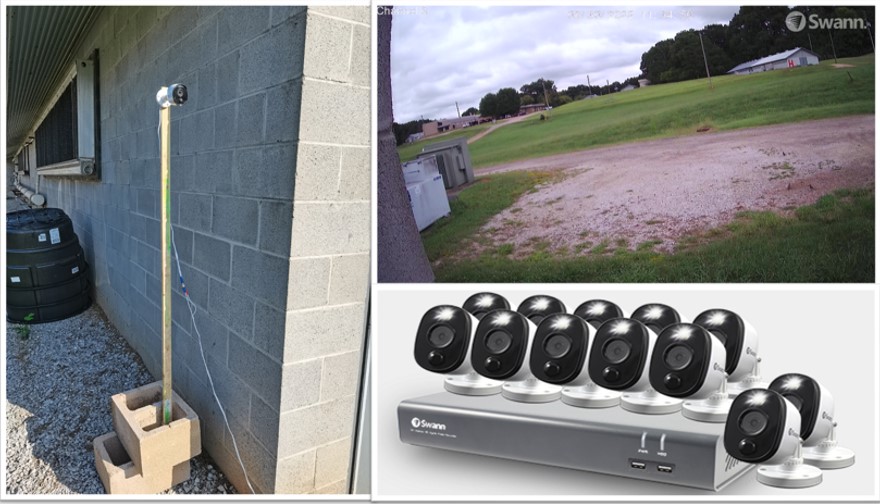
Figure 2. Wild birds monitoring system (cameras).
Two detection examples based on the two different models are shown (Figure 3 and Figure 4). Both can detect and locate Gadwall (a common reservoir of H5N1virus) and Brown Thrasher (a potential host of HPAI virus) correct. However, the detected confidence of YOLOX is higher than that of YOLOv5 (average higher 10%), which is also indirectly reflected that YOLOX has better accuracy. Nevertheless, models also generated some flaws when the body of wild birds occluded by the grass on the ground or other wild birds. It is a common error on object detection especially in an open filed area because there are a number of shelters and natural objects overlapping with target object. In addition, object size also affects detection accuracy. Wild birds such as Brown Thrasher have small size. To improve model performance of the small bird, high resolution images could be applied at prediction process. In this study, other errors coming from human interferes wild birds, which will alter wild birds away from the place we observe them. However, it could not be prevented because farm workers and researchers conducting experiments here have free access to their experimental houses. In addition, model performance is affected by weather conditions. Rainfall makes observing wild birds forceful because wild birds back to their shelters. However, even in sunny weather, there is also some limitations. The Sun’s light causes shadows, when shadows cover wild birds, which makes the detected target blur. To solve this issue, a dual-cue network is proposed to recover shadows in local pics. Region enhanced network with normalized attention is an alternative way to address shadows issue. In addition, deep metric leaning combines with knowledge graphs providing an efficient way to detect similar objects. Although, there are still some flaws in using automated detection system, the cornerstone (model accuracy) of it has reached acceptable result.
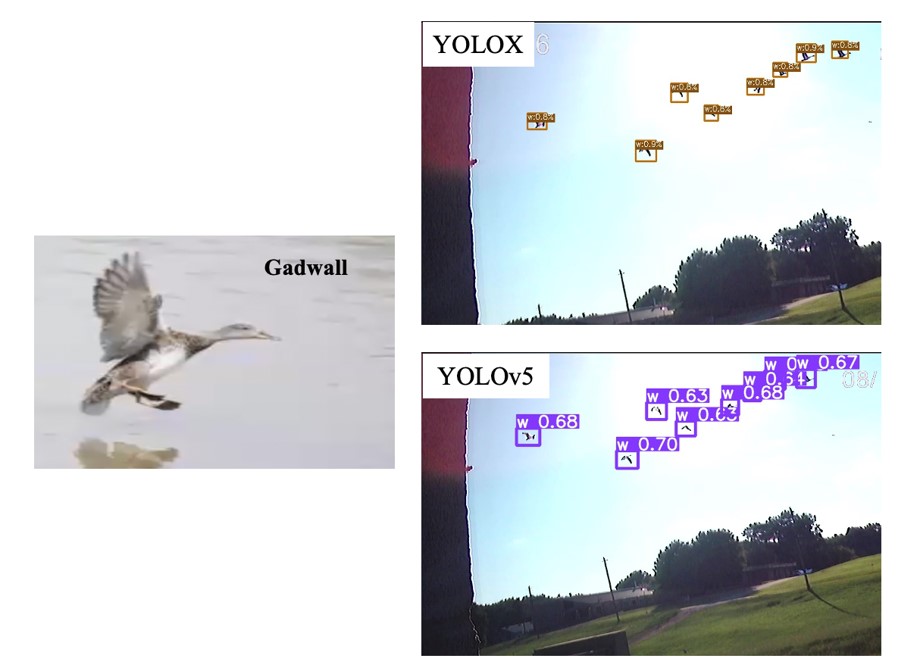
Figure 3. Detection of Gadwall by machine vision model automatically.
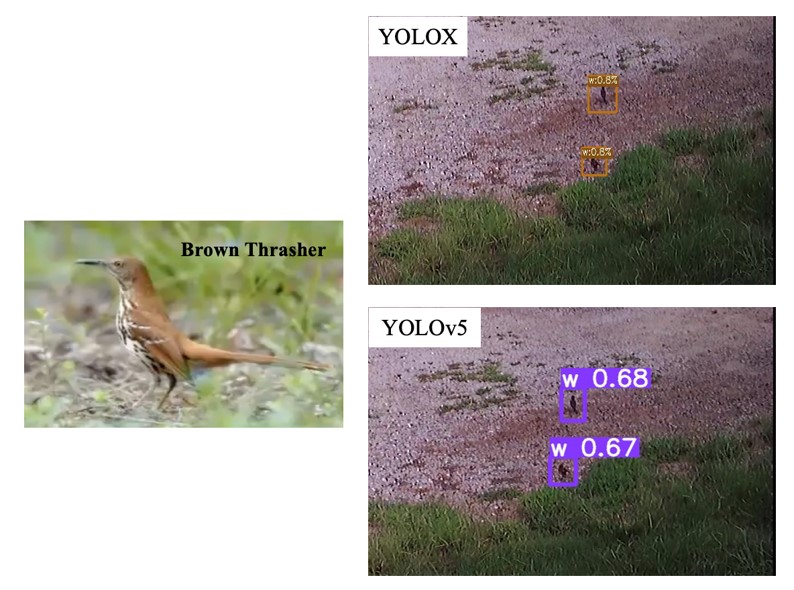
Figure 4. Detection of Brown Thrasher by machine vision model automatically.
This study compared two different deep learning algorithms, i.e., YOLOX and YOLOv5, to develop wild bird’s detection models. The overall accuracy of models ranges from of 86% to 95%, indicating that deep learning method is a useful approach to monitor wild birds. In addition, YOLOX is superior to YOLOv5 as it improves 9% accuracy using same training dataset and could be employed as the final model for wild birds’ detection. Some common wild birds (i.e., Gadwall and Brown Thrasher) can be recognized by our model, and their behavior patterns were conducted for applying in deterrent system to prevent AI transmission. This method plays a crucial role in developing a computer vision-based system to accurately locate potential wild birds that are reservoirs of AI viruses, which can reduce the risk of AI transmission from wild birds to poultry houses.
Further reading:
Yang, X., Bist, R. B., Subedi, S., Wu, Z., Liu, T., Paneru, B., & Chai, L*. (2024). A Machine Vision System for Monitoring Wild Birds on Poultry Farms to Prevent Avian Influenza. AgriEngineering, 6(4), 3704-3718. https://doi.org/10.3390/agriengineering6040211