The U.S. laying hen industry is transitioning from conventional caged (CC) systems to cage-free (CF) housing as driven by concerns of animal welfare, promoted by public demand for improved conditions and natural behavior among laying hens such as dustbathing (DB). The DB is a functionally important maintenance behavior in birds that clean plumage, realigns feather structures, removes feather lipids, which helps to remove parasites and prevents feathers from becoming too oily (Figure 1). For poultry housing, conventional caged egg production system has been criticized for welfare issues such as failure to provide hens to perform natural behaviors of DB. Researchers have looked at the lipid content of the feed used as a litter material and found that preference for a DB substrate increased with decreased lipid content at a threshold of 4% and above that threshold, preference was reduced dramatically. However, the litter conditions in CF houses tend to be wet or caked in winter due to reduced ventilation and accumulation of moisture. However, the manual detection of DB behavior is time-consuming, sometimes prone to errors, and have limitations. Therefore, an automated precision monitoring method is needed to detect DB behavior in laying hens from an early age in CF housing environment.
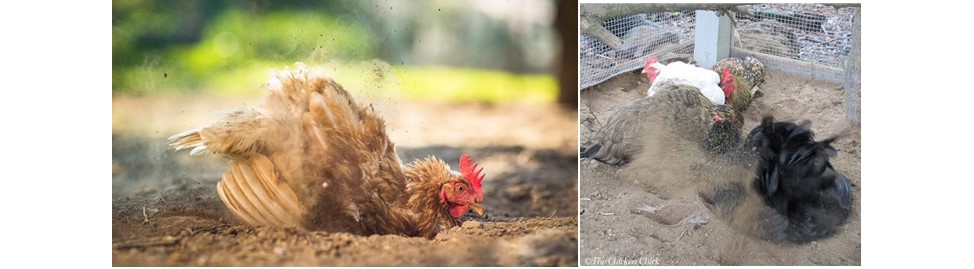
Fig. 1. Chickens’ dustbathing behavior (photo credit: The-Chicken-Chick.com).
To address DB monitoring challenges, researchers at the University of Georgia developed a deep learning-based imaging method (Figure 2 and Figure 3) for tracking DB automatically. This study was conducted in four identical research poultry facilities (rooms), each room housed about 200 Hy-line W-36 birds, at the University of Georgia, Athens, GA research facility. These rooms were designed with a provision of perches and litter floor (mixture of pine shaving at 2 inches depth, manure, and feathers, etc.).
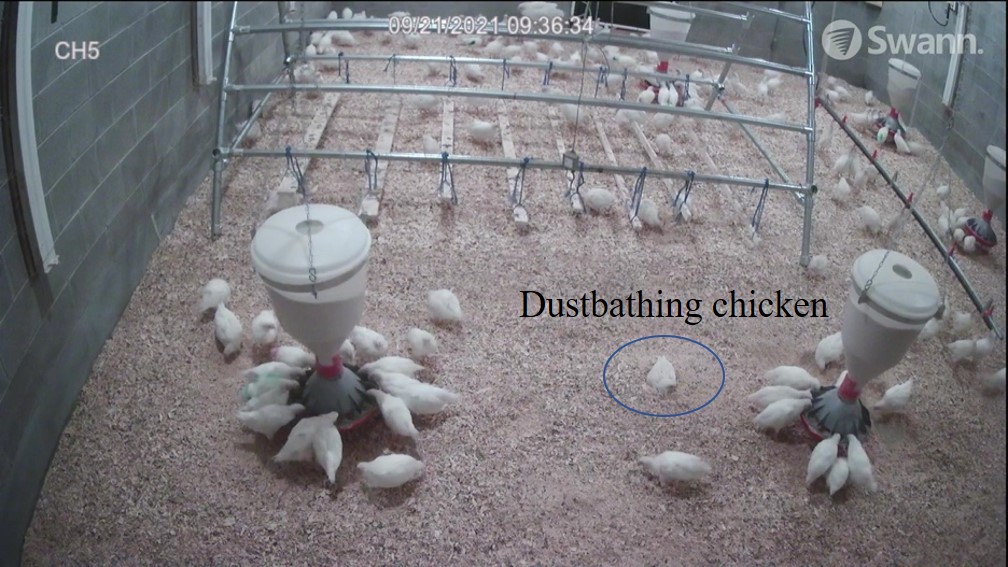
Fig. 2. Dustbathing behavior monitoring with imaging system.
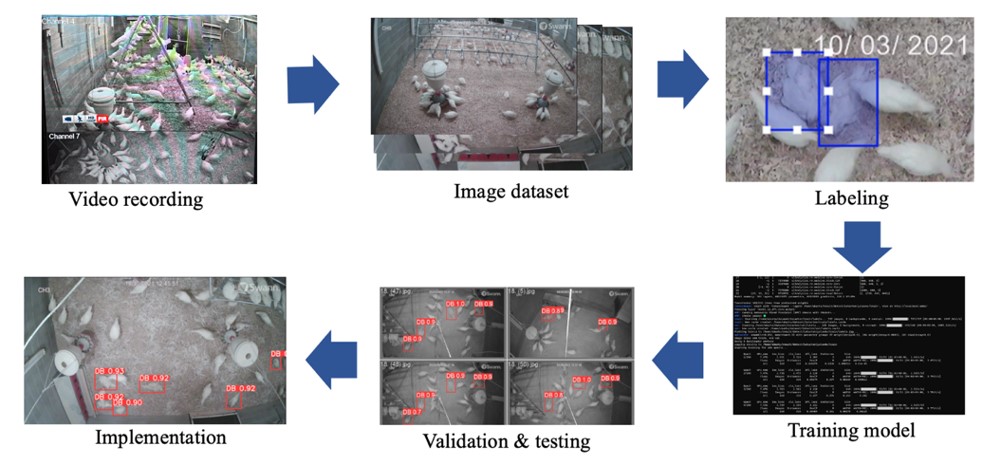
Figure 3. The processes of dustbathing detection system (i.e., data collection, labeling, training, validation, testing, and implementation).
In this study, deep learning models, i.e., YOLOv7-DB, YOLOv7x-DB, YOLOv8s-DB and YOLOv8x-DB, networks, were developed, trained, and compared in tracking DB behavior in 4 CF rooms each with 200 hens (W-36 Hy-Line). Results indicate that the YOLOv8x-DB model outperform all other models on detecting DB behavior with a precision of 93.4%, recall of 91.20%, and mean average precision (mAP@0.50) of 93.70% (Figure 4). All models performed with over 90% detection precision; however, model performance was affected by equipment like drinking lines, perches, and feeders. Based on the optimal model (YOLOv8x-DB), DB detection precision was highest during grower phase (precision of 96.80%, recall of 97.10%, mAP@0.50 of 98.60%, and mAP@0.50-0.95 of 79.10% followed by pre-lay, layers, developer, and peaking phases. This study provides a reference for poultry and egg producers that DB behavior can be detected automatically with precision of at least 89% or more using optimal model at any growing phase of laying hens.
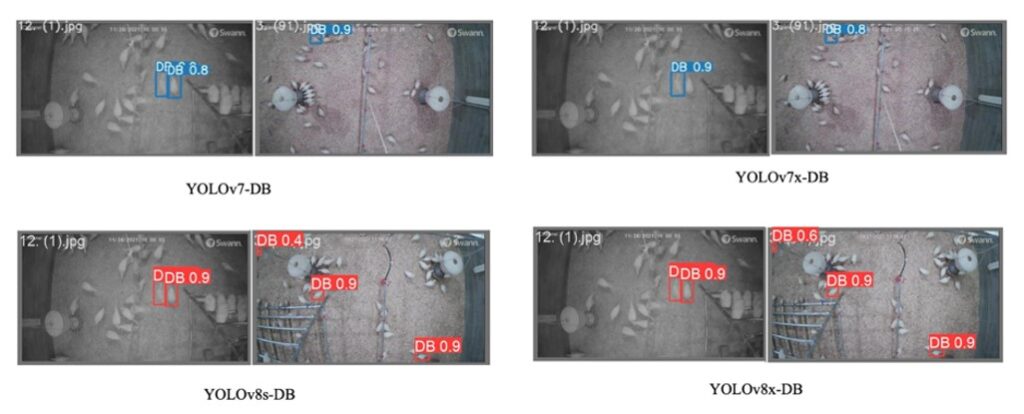
Figure 4. Comparison of detected dustbathing behavior (DB) among various YOLOv7-DB and YOLOv8-DB models.
This study provides a comprehensive DB detection analysis, including valuable insights and performance metrics of different YOLOv7-DB and YOLO8-DB models. Despite the challenges posed by variables like the presence of drinkers, feeders, and dust, the YOLOv8x-DB model outperformed all other models in accurately detecting DB behavior. The YOLOv8x-DB model resulted in higher precision, recall, mAP, and F1 scores for detecting DB behavior in CF housing conditions and provides its ability and reliability than other models although all other models also resulted in precision of at least 90% or more in detecting DB behavior. This study highlighted the benefits of utilizing the latest addition of CNN deep learning models, i.e. YOLOv8x in tracking DB behavior. This finding can provide a valuable reference for developing a tool for detecting DB behavior in cage-free production systems.
Further reading:
Paneru, B., Bist, R., Yang, X., & Chai, L*. (2024). Tracking dustbathing behavior of cage-free laying hens with machine vision technologies. Poultry Science, 103(12), 104289.