Footpad dermatitis (FPD) is a common poultry condition that can negatively influence chickens’ production, welfare, and health. FPD also called pododermatitis, is a widespread condition affecting broilers and laying hens. It is characterized by the development of inflammatory lesions, ulcers, and necrotic tissue on the plantar surface of the chickens’ feet. The FPD condition is often influenced by a combination of environmental factors (e.g., litter quality), stocking density, and overall health management. The FPD lesions can cause pain and discomfort in affected birds, impacting their walking and access to feed and water resources. Such challenges could further lead to reduced body weight, decreased egg production, and heightened veterinary expenditures. The FPD could FPD could happen for 50% or higher percentage of chickens for poorly managed flock and is severely devaluating the billion-dollar poultry industry in the USA (9 billion chickens at farm gate value of $35 billion in 2023) and the world.
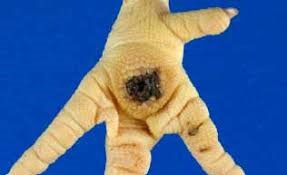
Fig. 1. Footpad dermatitis in broiler chicken (photo credit: Aviagen).
To address FPD monitoring challenges, researchers at the University of Georgia developed a machine vision-based method (Figure 2) for scoring footpad score automatically. Early FPD assessment can also help with timely intervention and reinforce consumer confidence in buying welfare-labeled products. The gold standard manual FPD assessment could be time-consuming and laborious and need skilled welfare assessors, which could limit labor shortages in farm work. Computer vision for data collection and machine learning for data analysis offer an alternative efficient solution for FPD scoring, which can overcome the abovementioned manual assessment drawbacks.
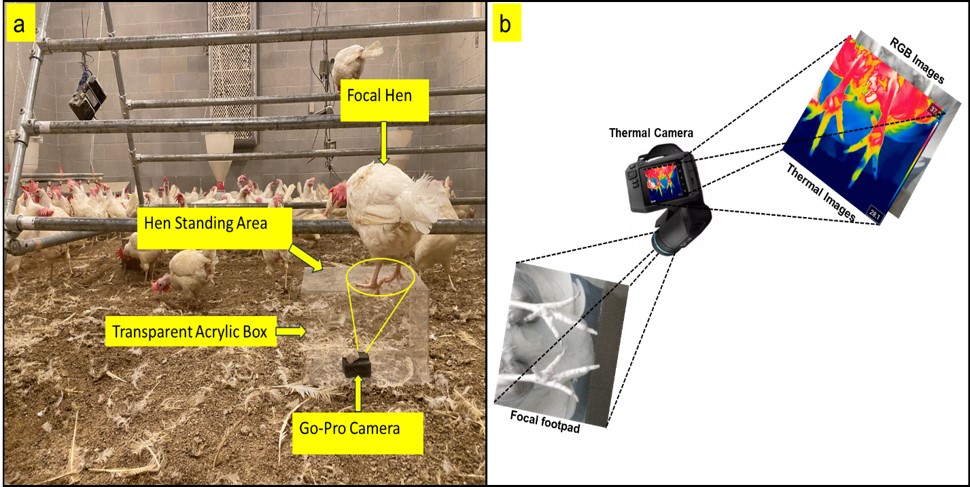
Fig. 2. Footpad dermatitis conditions recording set up in cage-free hen housing using a) GoPro camera and b) Thermal camera.
In this study, the YOLOv7 and YOLOv8 models (Figure 3) were extracted from GitHub Ultralytics for analyzing footpad scores. The system was tested in a research cage-free facility at UGA’s research farm. A total of 700 Hy-Line W-36 hens were raised in four cage-free housing systems integrated with Electrostatic Particle Ionization and various bedding materials. A GoPro camera with an upward lens was placed inside a transparent box. Individual laying hens were placed on the top surface of the box to acquire RGB images. In addition, a thermal camera was used to record RGB and thermal images of footpads, and the images were manually scored to assess their footpad conditions. Preprocessing techniques (e.g., filtration, separation, and augmentation) were deployed to enhance dataset quality and size. Moreover, YOLOv8 models (YOLOv8n, YOLOv8s, YOLOv8m, YOLOv8l, and YOLOv8x) and YOLOv7 models (YOLOv7 and YOLOv7x) were comparatively evaluated for predicting FPD scores. From the tested images, comprehensive analysis confirmed that remarkable performance of the “YOLOv8l-FPD” model in effectively identifying FPD instances (Fig. 3). The model’s higher recall and mAP scores collectively underscore its proficiency in recognizing FPD instances across diverse scenarios (Figure 4). Each incremental improvement in performance metrics was important in reducing false detections by the model.
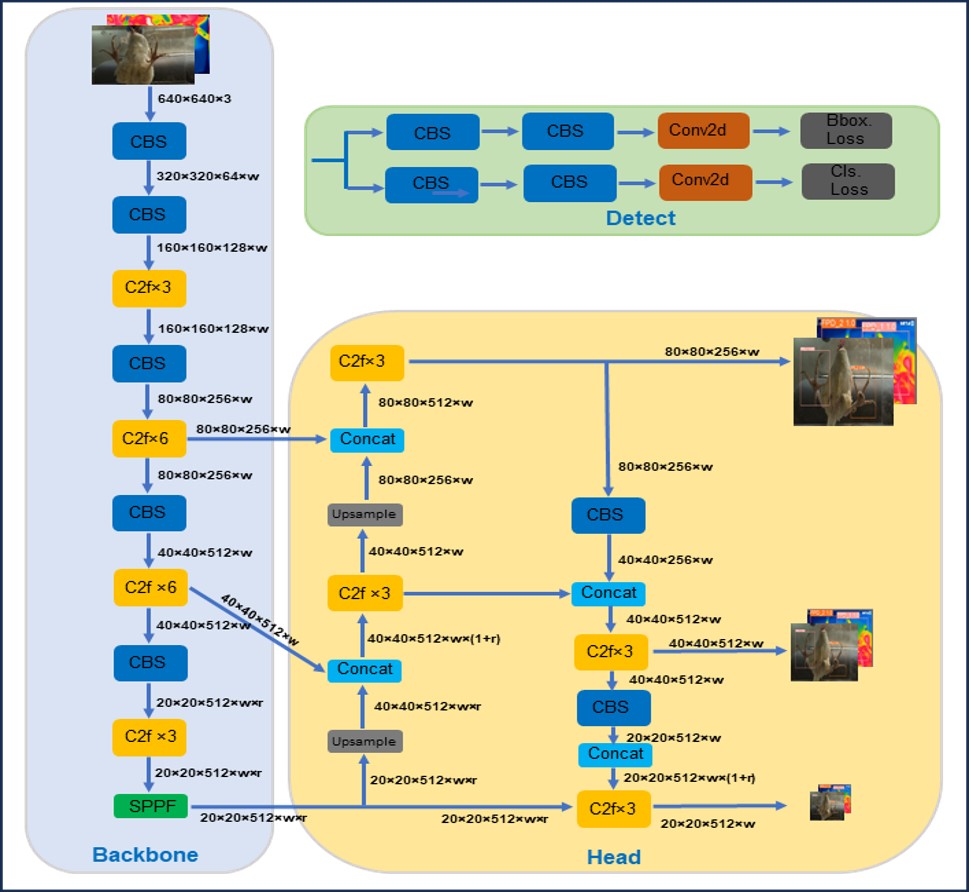
Fig. 3. Architecture of the YOLOv8 model for FPD scoring and detection. Where, C2f- Cross-Stage Partial Bottleneck with two Convolutions; CBS- composed of convolution (Conv2d), batch normalization, and SiLu activation functions; SPPF- adopted Spatial Pyramid Pooling (SPP) fast with fewer FLOPs.
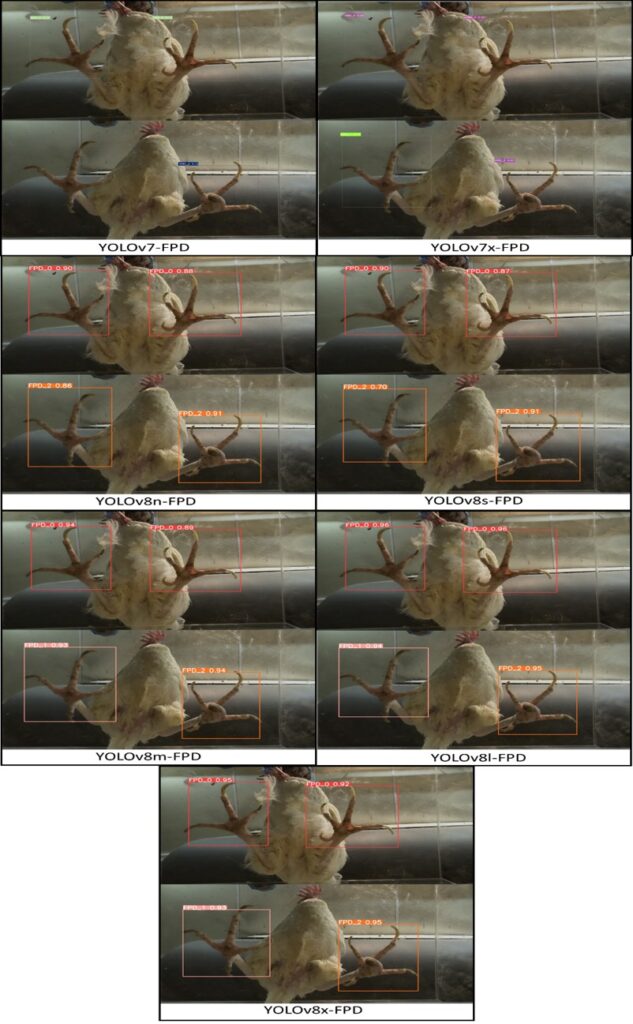
Fig. 4. Comparison of detected footpad dermatitis score across various models in test datasets.
Overall, the YOLOv8l-FPDThermal model comparably demonstrated higher performance metrics throughout most of the training process. This implies that utilizing thermal images for training yields enhanced accuracy in detecting and scoring FPD (Fig. 5). From inception, the slightly higher performance of the YOLOv8l-FPDThermal model underscores the intrinsic advantages of thermal imagery in fostering more accurate and reliable FPD detection.
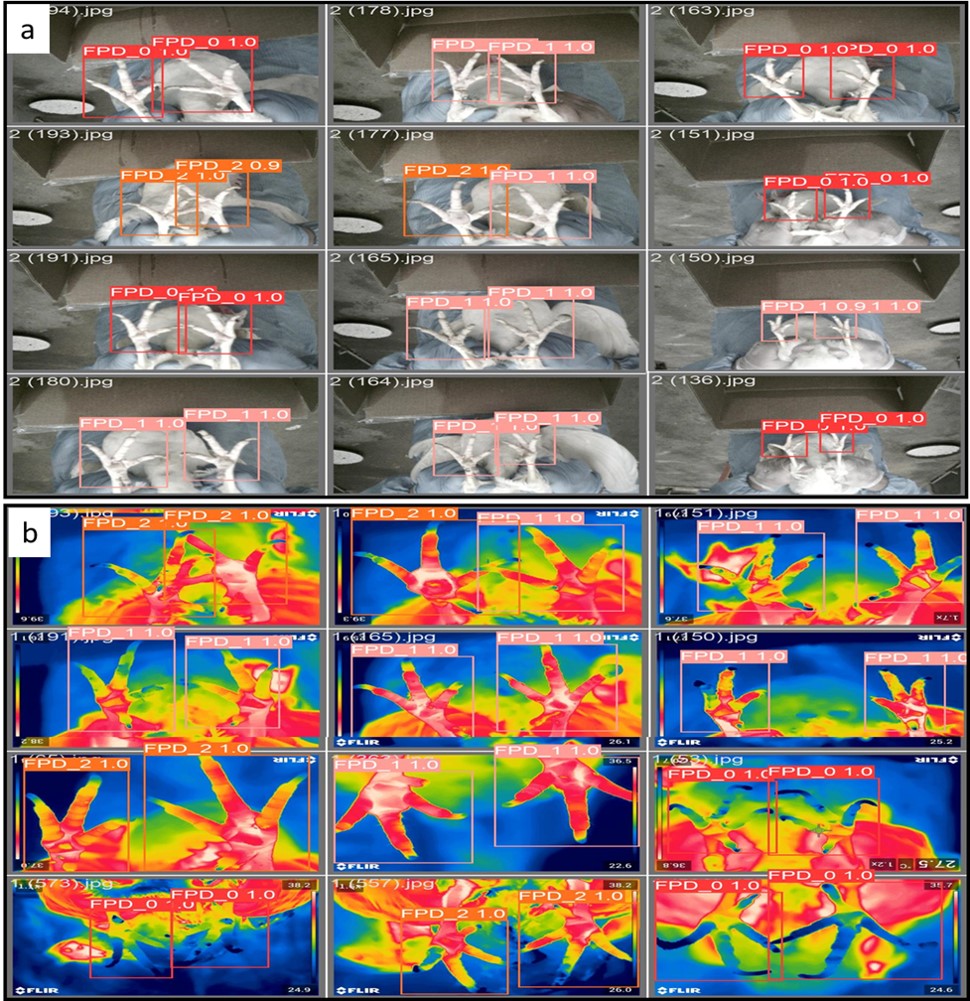
Fig. 5. The FPD score detected in test image datasets using the YOLOv8l model for a) RGB images and b) thermal images.
In this study, the newly trained model YOLOv8l outperformed other models, with higher recall (96.6%), mAP@0.50 (97.0%), and F1-score (95.0%). Additionally, the YOLOv8l-FPD model exhibited a high mAP@0.50 for score 0 (98.0%), score 1 (95.0%), and score 2 (97.9%) and F1-score (95.0%) for all FPD scores. Using thermal images could result in faster convergence of model training and slightly better FPD score prediction performance than RGB images. The proposed technique can be useful for non-invasive automatic FPD scoring and further improve automation levels and animal welfare in the egg industry.
Further reading:
Bist, R. B., Yang, X., Subedi, S., Bist, K., Paneru, B., Li, G., & Chai, L.* (2024). An automatic method for scoring poultry footpad dermatitis with deep learning and thermal imaging. Computers and Electronics in Agriculture, 226, 109481.