Introduction
While CF house allows hens to perform more natural behaviors (e.g., dust bathing, perching and foraging on the litter floor), a particular challenge is floor eggs (i.e., mislaid eggs on litter floor). Floor eggs have high chances of contamination. The manual collection of eggs is laborious and time-consuming. Delayed floor egg collection may lead to eggs broken or eaten by other birds. Prior to addressing the floor egg issues, it is challenging for egg producers to transit from conventional cage systems to CF productions in EU and USA. Therefore, precision poultry farming technology is necessary to detect floor eggs.
Methods
Researchers in the Department of Poultry Science at the University of Georgia conducted the experiment on poultry research farm. A total of 800 W36 Hy-Line hens were raised in four research cage-free facilities (200 birds in each room from day 1), each measuring 7.3 m long × 6.1 m wide × 3 m high. Pine shavings were uniformly spread on the floor (5 cm depth) before bird arrival, and commercial feed was provided ad libitum. Commercial layer management guidelines for Hy-Line W-36 were used to mange the flock. The Institutional Animal Care and Use Committee (IACUC) of the University of Georgia, USA, approved animal use and management.
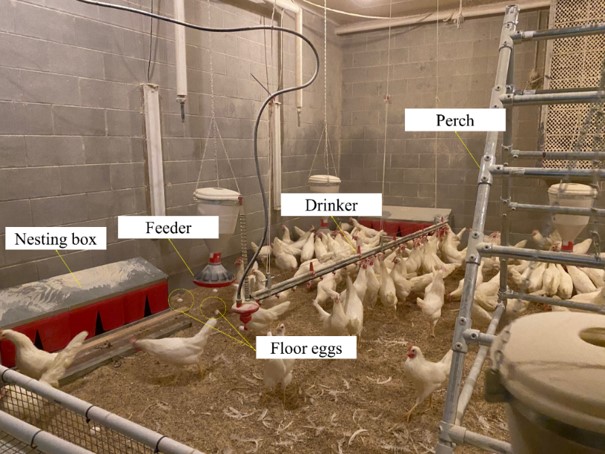
Figure 1. The research cage-free facility (200 hens per room; 7.3 m L × 6.1 m W × 3 m H).
Data collection and preparation
Six night-vision network cameras (PRO-1080MSB, Swann Communications USA Inc., Santa Fe Springs, LA, USA) were mounted above the drinking system, feeders, and perches and on the wall at ~3 m above the ground to capture top-view videos and footage from sideways. In addition, different areas of eggs laid and hens’ activities were continuously monitored, and videos were stored in digital video recorders (DVR-4580, Swann Communications USA Inc., Santa Fe Springs, LA, USA). The video files (.avi format) were recorded with a resolution of 1920 × 1080 pixels at a sample rate of 15 frames per second (fps) and converted to image files (.jpg) using Free Video to JPG Converter (ver. 5.0).
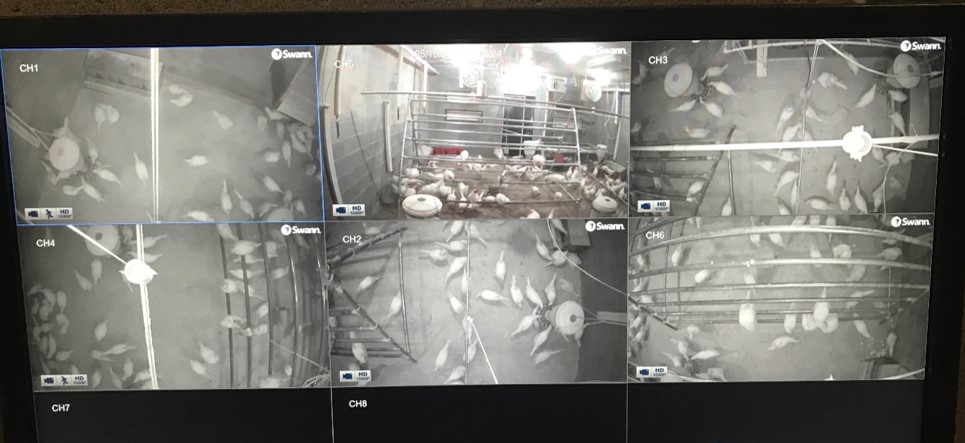
Figure 2. Imaging system and data collection.
Results
The optimized model, after training, was used to detect the floor eggs in new unlabeled images. The Figures 3 & 4 show some examples of the automatic detection of floor eggs. The YOLOv5s-egg, the YOLOv5x-egg, and the YOLOv7-egg models were compared with the following observations: (1) the YOLOv5s-egg model can detect the eggs with a precision of 87.9%, recall of 86.8%, and mean average precision (mAP) of 90.9%; (2) the YOLOv5x-egg model can detect the eggs with a precision of 90%, recall of 87.9%, and mean average precision (mAP) of 92.1 %; (3) the YOLOv7-egg model can detect the eggs with a precision of 89.5%, recall of 85.4%, and mean average precision (mAP) of 88%; and 4) the YOLOv5x-egg model detected floor eggs with higher accuracy, precision, mAP, and recall than YOLOv5s-egg and YOLOv7-egg.
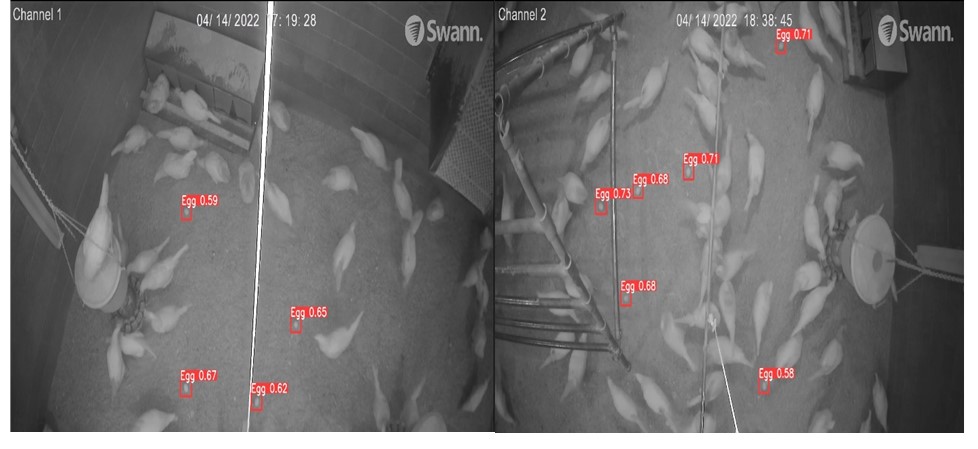
Figure 3. Performance of all three models in floor eggs (UGA farm).
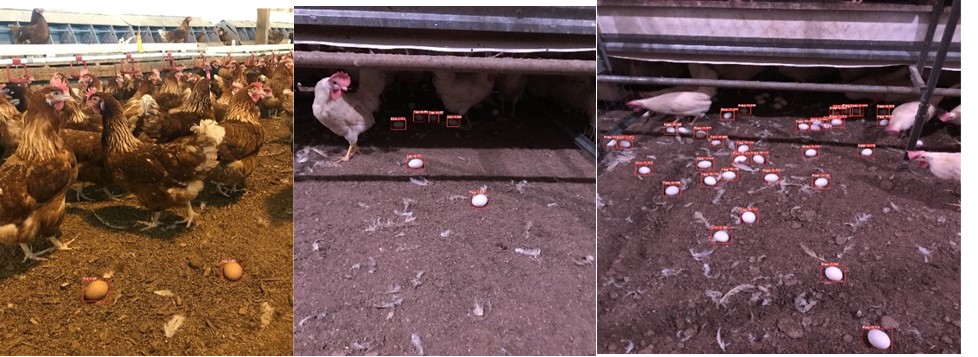
Figure 4. Performance of three models in floor houses (Commercial farms: left – organic farm in the USA; middle and right – cage-free farm in the USA).
Summary
In this study, three new deep learning models, i.e., YOLOv5s-egg, YOLOv5x-egg, and YOLOv7-egg networks, were developed, trained, and compared in tracking floor eggs in four cage-free laying hen research facilities. Models were verified to detect eggs by using images collected from two different commercial houses. All models perform well in precision and detection; however, model performance is affected by the stocking density, varying light intensity, and images occluded by equipment like drinking lines, perches, and feeders. The YOLOv5x-egg model detected floor eggs with higher accuracy, precision, mAP, and recall than YOLOv5s-egg and YOLOv7-egg. This study provides a reference for cage-free producers that floor eggs can be monitored automatically. Future studies are guaranteed to test the system in commercial houses. Furthermore, the detection of floor eggs as an object with the help of deep learning and object detection can be used for developing a robotic egg-picking system. As the commercial house is about 150 m long and 40-50 m wide, it is suggested to use mobile imaging system for collecting images from different locations periodically at a specific high. In addition, an APP will be developed for tracking results of floor eggs.
Further reading:
Subedi, S., Bist, R.B, Yang, X., Chai, L (2023). Tracking Floor Eggs with Machine Vision in Cage-free Hen Houses. Poultry Science, 102637. https://doi.org/10.1016/j.psj.2023.102637