Defective eggs (eggs with abnormal exterior quality and size, Figure 1) can reduce the egg quality/food safety and market value of laying hen production, especially in cage-free houses where floor eggs are more common. To improve egg quality, automated grading and defect detection systems have been developed using machine vision and image processing. Automated egg measurement systems are also used to sort eggs by weight, ensuring maximum market value. Previous studies primarily focused on using computer vision techniques for egg classification, but few have combined deep learning and machine vision techniques for joint egg classification and weighting.
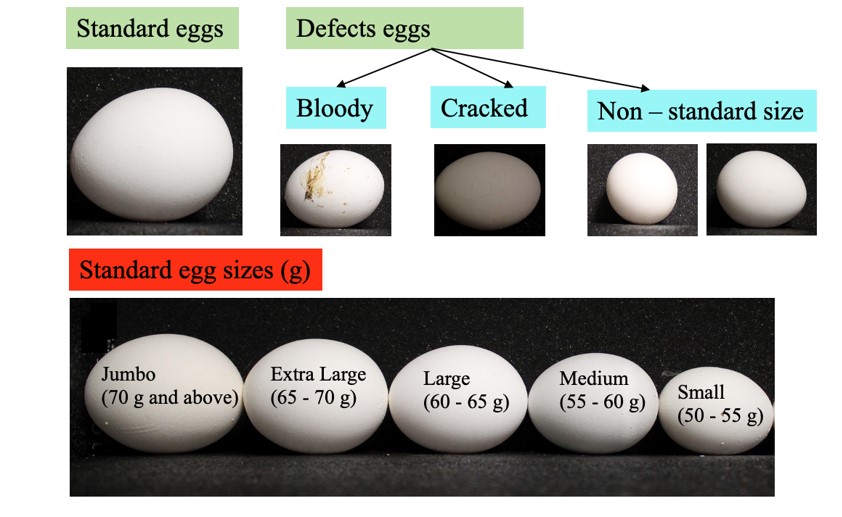
Figure 1. Egg exterior quality and size.
To address this gap, researchers at the University of Georgia’s poultry science department developed a two-stage model based on real-time multitask detection (RTMDet) and random forest networks for predicting egg category and weight (Figure 2). The egg weight information was obtained by an egg imaging system (Figure 3) that is consisted of a camera; a tripod; a egg base; a computer; and a digital scale.
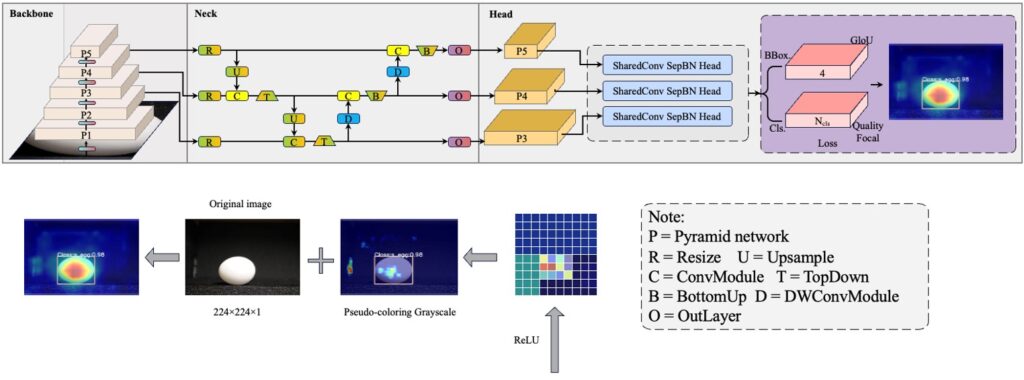
Figure 2. The structure of egg classification based on RTMDet.
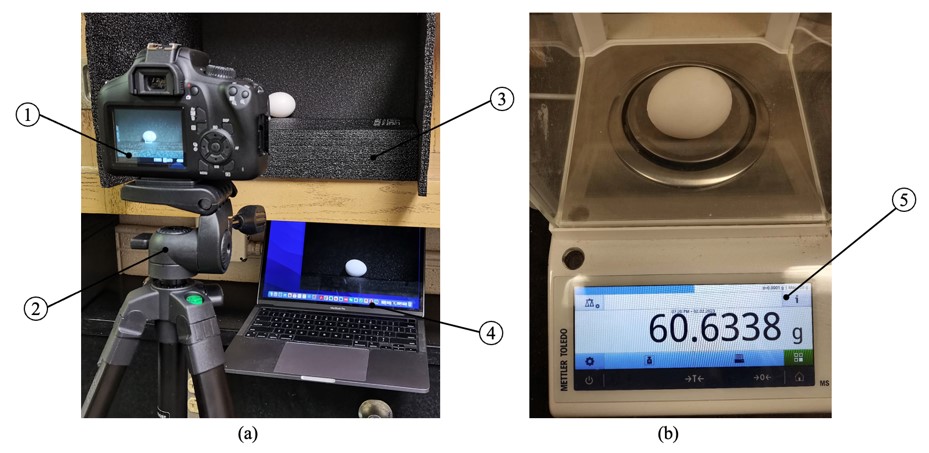
Figure 3. The egg imaging system for classifying eggs (a) and weighting eggs (b).
The model uses convolutional neural network (CNN) and regression techniques to perform joint egg classification and weighing. RTMDet was used to sort and extract egg features for classification, and a Random Forest algorithm is used to predict egg weight based on the extracted features (major axis and minor axis). The new models can predict bloody eggs, cracked eggs, jumbo eggs, and different other defects (Figure 4) and eight weight (Figure 5).
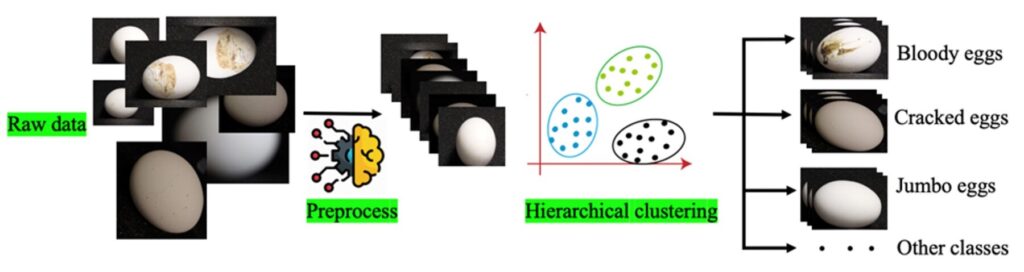
Figure 4. The flow of egg data processing.
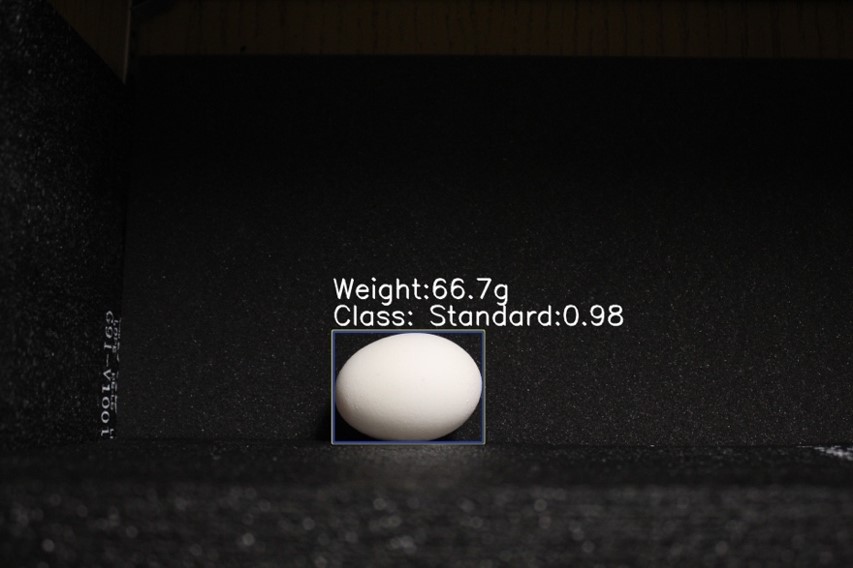
Figure 5. The egg has been classified as ‘Standard’ and its predicted weight of 66.7 g.
The results of the study showed that the best accuracy achieved was 94.8% and best R2 is 96.0%. In addition, the model can be used to automatically exclude non-standard size eggs and eggs with exterior issues (e.g., calcium deposit, stains, and cracks). This detector is among the first models that perform the joint function of egg sorting and weighing eggs, and is capable of classifying them into different categories (i.e., crack, bloody, and non-standard size). By implementing the findings of this study, the egg producers can reduce costs and increase productivity, ultimately leading to enhanced egg quality and safe products for consumers.
Further reading:
Yang, X., Bist, R. B., Subedi, S., & Chai, L. (2023). A computer vision-based automatic system for egg grading and defect detection. Animals, 13(14), 2354. https://doi.org/10.3390/ani13142354