Animal activities and behaviors provide significant insights into the mental and physical well-being of poultry, serving as a key indicator of their health and subjective states. For instance, predicting indoor particulate matter concentration in poultry houses can be informed by broiler activity and ventilation rates. Additionally, locomotor activity acts as a proxy for the gait of individual broilers, facilitating gait classification. Currently, such activity is primarily monitored through manual methods, which are labor-intensive and prone to individual bias. With the world’s population projected to reach 9.5 billion by 2050 and the demand for animal products like meat, eggs, and milk expected to increase by 70% from 2005 levels, developing automated, precise systems for monitoring poultry activity becomes crucial. This advancement is especially important for managing health and welfare efficiently under the constraints of limited natural resources such as fresh water, feed, and land. Automated measurement systems are increasingly vital for monitoring and promoting good welfare within the growing livestock industry. Over recent decades, advancements in digital imaging technologies have spurred the development of automated and precise animal activity tracking systems.
Researchers at the University of Georgia developed a poultry flock activity and behavior tracker (Figure 1) using deep learning models. They compared the performance of popular CNN models, including YOLOv5, YOLOv8, ByteTrack, DeepSORT, and StrongSORT in tracking chickens’ behaviors; and identified the best model’s performance in potential applications such as detecting piling and smothering behaviors, as well as monitoring footpad health based on flock activity. Six individual experiments on chicken tracking were conducted to discover the optimal tracker for monitoring birds’ activity. The evaluation compared models based on the YOLO architecture, enhanced with tracking algorithms such as DeepSORT, StrongSORT, and ByteTrack. The YOLOv5 models, combined with DeepSORT, StrongSORT, and ByteTrack, displayed commendable results. The YOLOv5+DeepSORT model achieved a MOTA of 80%, an IDF1 score of 78%, processed at 25 FPS, and recorded 50 identity switches. YOLOv5+StrongSORT improved these results with a MOTA of 85%, an IDF1 score of 83%, fewer identity switches 35, and a slightly lower FPS of 22. The integration of ByteTrack further adjusted the trade-off, with YOLOv5+ByteTrack exhibiting an 83% MOTA, 81% IDF1, 40 identity switches, and the highest FPS of 28 among the YOLOv5 series.
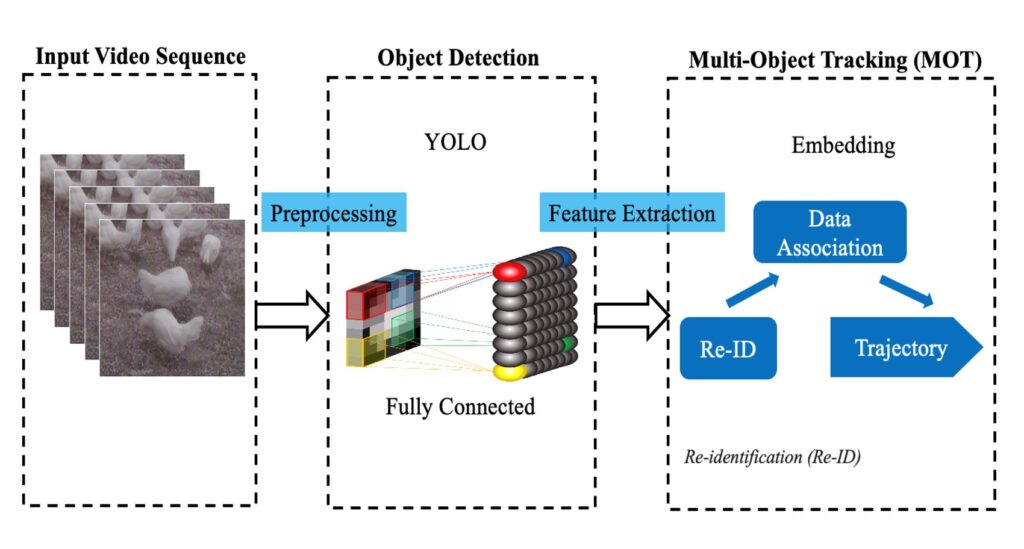
Figure 1. Chicken activity and behavior tracker based on multi-object tracking.
To rigorously evaluate the advanced YOLOv8+DeepSORT model’s capability in monitoring cage-free poultry environments, we conducted an analysis using a 73-second video to ascertain the model’s efficacy in determining the average distance among the flock. In this video, the model successfully identified a maximum of 91 chickens. During the initial 49 seconds of the footage, the chickens exhibited aggregation behavior, resulting in an increasing average distance. After this period, most of the chickens moved out of the camera’s field of view. The remaining chickens distributed near corners or feeders, showed a decrease in average distance. This dynamic was captured in visualized results, and corresponding images highlighting detection overlays are presented in Figure 2.
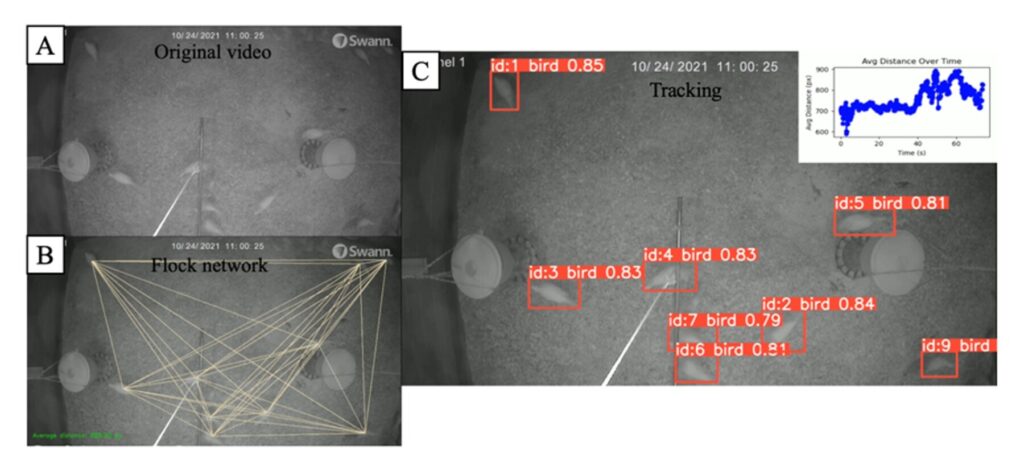
Figure 2. Integrated approach to poultry behavior study: original video (a), flock network analysis (b), and individual tracking with temporal distance data (c).
Piling and smothering are abnormal behaviors in cage-free laying hens, where piling happens when birds crowd together, and smothering is the serious condition that results from long-term crowding, often leading to the death of chickens. Therefore, the new method was applied to monitor piling and smothering behaviors. We found that chickens piled up due to their fear of unfamiliar people (Figure 3A), and they also gathered in preferred resting places at night (Figure 3B). Under these two piling and smothering behaviors, the average distance between chickens is 255.99 pixels and 456.78 pixels, respectively, which is at least 28.82% lower than in normal situations, which means the range of average distance detection within a chicken flock is between 641.72 and 892.95 pixels, as discussed in part 3.2. We used the minimum value, 641.72 pixels, to compare with 456.78 pixels and found it to be 28.82% lower, based on our 73-second continuous monitoring of normal activities. Additionally, when piling and smothering occur in only a portion of the camera view, we can use region-based detection to identify these behaviors in high-risk areas like side walls, doors, and nesting boxes. Moreover, in the absence of clear fear-inducing elements in the camera footage, environmental data can be combined to investigate potential causes of piling and smothering. According to the Hy-Line W-36 Commercial Layers Management Guide, chicks are prone to cluster together in response to cold temperatures. Additionally, uneven ventilation or light distribution within the enclosure may prompt chicks to congregate in specific areas, seeking to avoid drafts or noise.
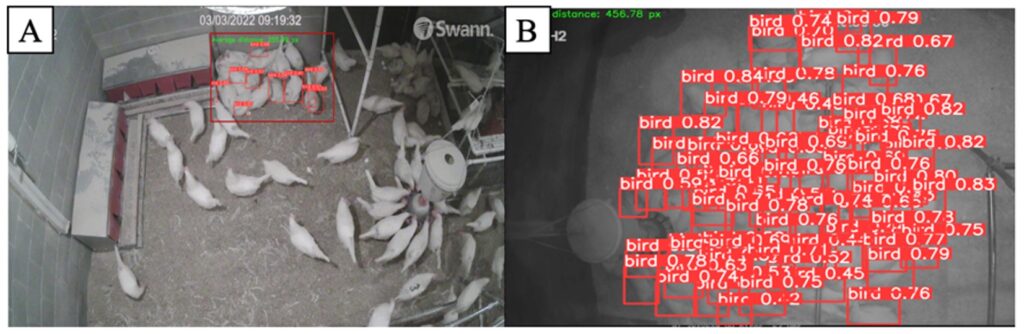
Figure 3. The detection of piling behavior in cage-free houses: A shows piling due to stressful conditions, while B depicts piling resulting from birds gathering in a preferred location.
Further reading: Yang, X., Bist, R.B., Paneru, B., and Chai, L. (2024). Monitoring Activity Index and Behaviors of Cage-free Hens with Advanced Deep Learning Technologies. Poultry Science, 104193. https://doi.org/10.1016/j.psj.2024.104193