In recent years, artificial intelligence (AI) advancements have greatly influenced the agricultural industry, particularly with the emergence of large foundation models. One such model, the Segment Anything Model (SAM) developed by Meta AI Research, has revolutionized object segmentation tasks. SAM has a revolutionary object segmentation utility by employing a cutting-edge foundation model. SAM demonstrates extraordinary zero-shot segmentation capabilities by supporting visual cues such as points, boxes, and masks. This is due to its extensive data training. In contrast to conventional models, SAM’s distinct prompt capability makes it highly versatile and accurate in object segmentation, which has applications in numerous fields, including agriculture. It enables researchers and practitioners to enhance detection of pests and leaf diseases, as well as crop segmentation. While SAM has demonstrated success in various agricultural applications, its potential in the poultry industry, specifically regarding cage-free hens, remains largely unexplored.
In a recent study led by researchers at the University of Georgia, a Meta AI Research model SAM was used to build a new tracking method for monitoring individual chickens for behavior and activity analysis (Figure 1).
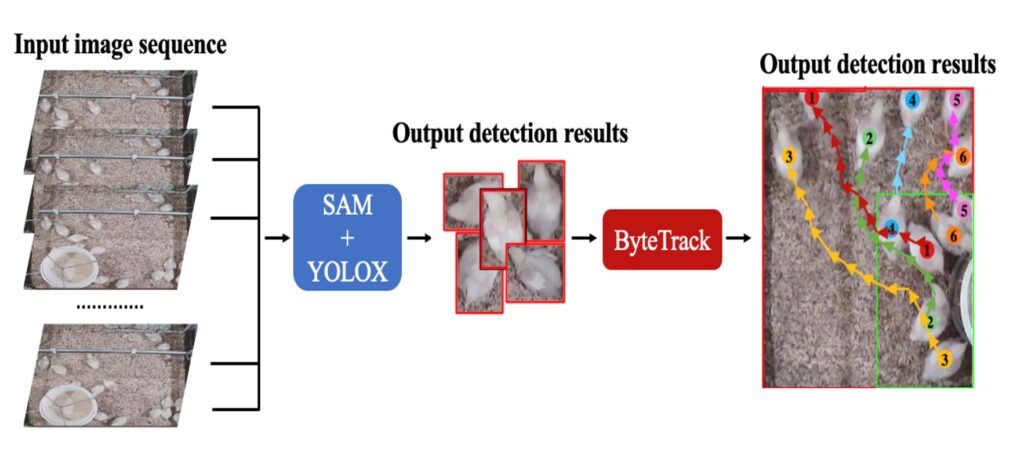
Figure 1. SAM-based poultry tracking method.
This study aimed to evaluate SAM’s zero-shot segmentation performance for chicken segmentation tasks, including part-based segmentation and the utilization of infrared thermal images. Additionally, it investigated SAM’s ability to predict weight and track chickens. The results highlight SAM’s superior performance compared to SegFormer and SETR for both whole and part-based chicken segmentation. SAM demonstrated remarkable performance improvements, achieving a mean intersection of union (mIoU) of 94.8% when using the total points prompts. Furthermore, SAM-based chicken segmentation provides valuable insights into weight prediction, as well as behavior and movement patterns of broiler birds. These findings contribute to the understanding of SAM’s potential in poultry science, paving the way for future advancements in chicken segmentation and tracking using large foundation model.
The impact of flock density on the SAM’s accuracy was assessed by testing its performance under varying levels of flock density. Three distinct density categories were established: low density (0–5 birds/m² with 95.60% accuracy), moderate density (5–9 birds/m² with 95.58% accuracy), and high density (9–18 birds/m² with 60.74% accuracy). These categories provided a comprehensive framework for evaluating the model’s effectiveness across different flock concentrations. SAM encounters difficulties in segmenting individual chickens when flock density surpasses 9 birds/m2. The overlapping bodies of chickens pose a significant challenge for the model in accurately distinguishing and segmenting each bird. This limitation becomes more pronounced in regions with high flock density, as highlighted in green circle in Figure 2.
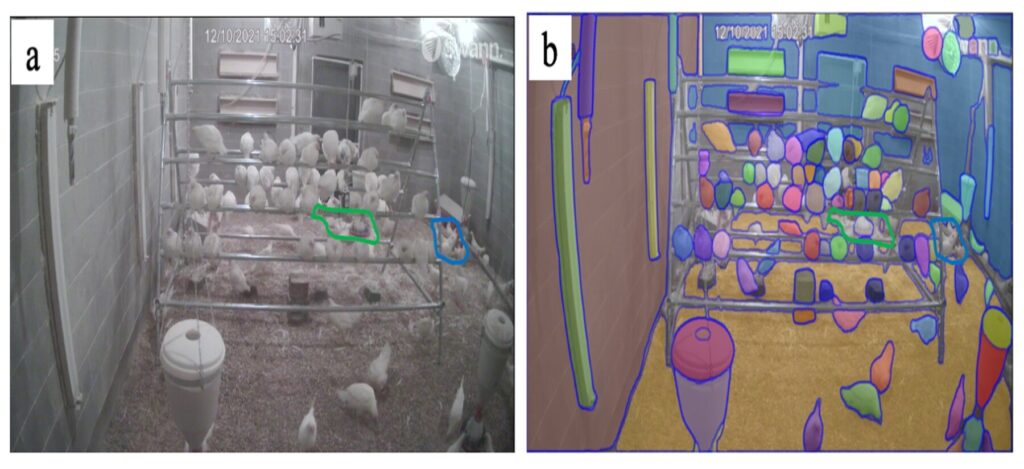
Figure 2. Side view of chicken segmentation in a research cage-free house (a, b).
In this study, researchers identified five basic behaviors: feeding (characterized by the head positioned above the feeder), drinking (with the head near the nipples), perching (feet gripping a wooden stud), walking (body movement across the litter), and dust bathing (the body rolling in the litter). SAM faces challenges in accurately detecting chickens when they display various behaviors, resulting in changes in their body postures. For instance, in the red rectangle emphasized in Figure 3, a chicken is observed roosting with its head nestled inside its feathers and its body adopting a more compact shape, deviating from the usual streamlined body posture. Due to the lack of specific training in this domain, SAM erroneously identifies the roosting chicken as two distinct individuals instead of recognizing its actual state.
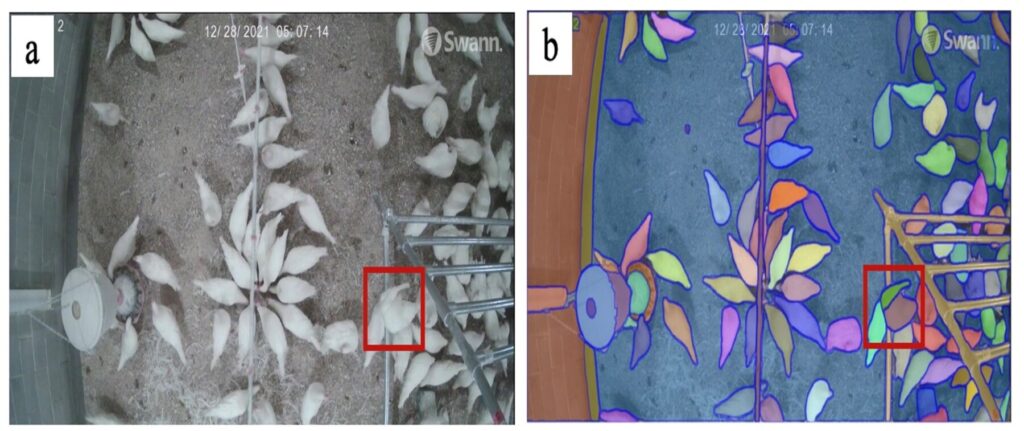
Figure 3. Top view of chicken segmentation in a research cage-free house (a, b).
This study presents the development and evaluation of SAM, a novel computer vision model for chicken segmentation, weight prediction, and tracking. The findings demonstrate SAM’s superiority over existing methods in both semantic and part-based segmentation. Moreover, the combination of SAM with RF proves promising for chicken weight prediction, while integrating SAM with YOLOX and Byte-Tracker enables real-time tracking of individual broiler bird movements. This work was among the first to use large language model for precision poultry production.
Further reading:
Yang, X., Dai, H., Wu, Z., Bist, R.B., Subedi, S., Sun, J., Lu, G., Li, C., Liu, T. and Chai, L. (2024). An innovative segment anything model for precision poultry monitoring. Computers and Electronics in Agriculture, 222, 109045. https://doi.org/10.1016/j.compag.2024.109045